
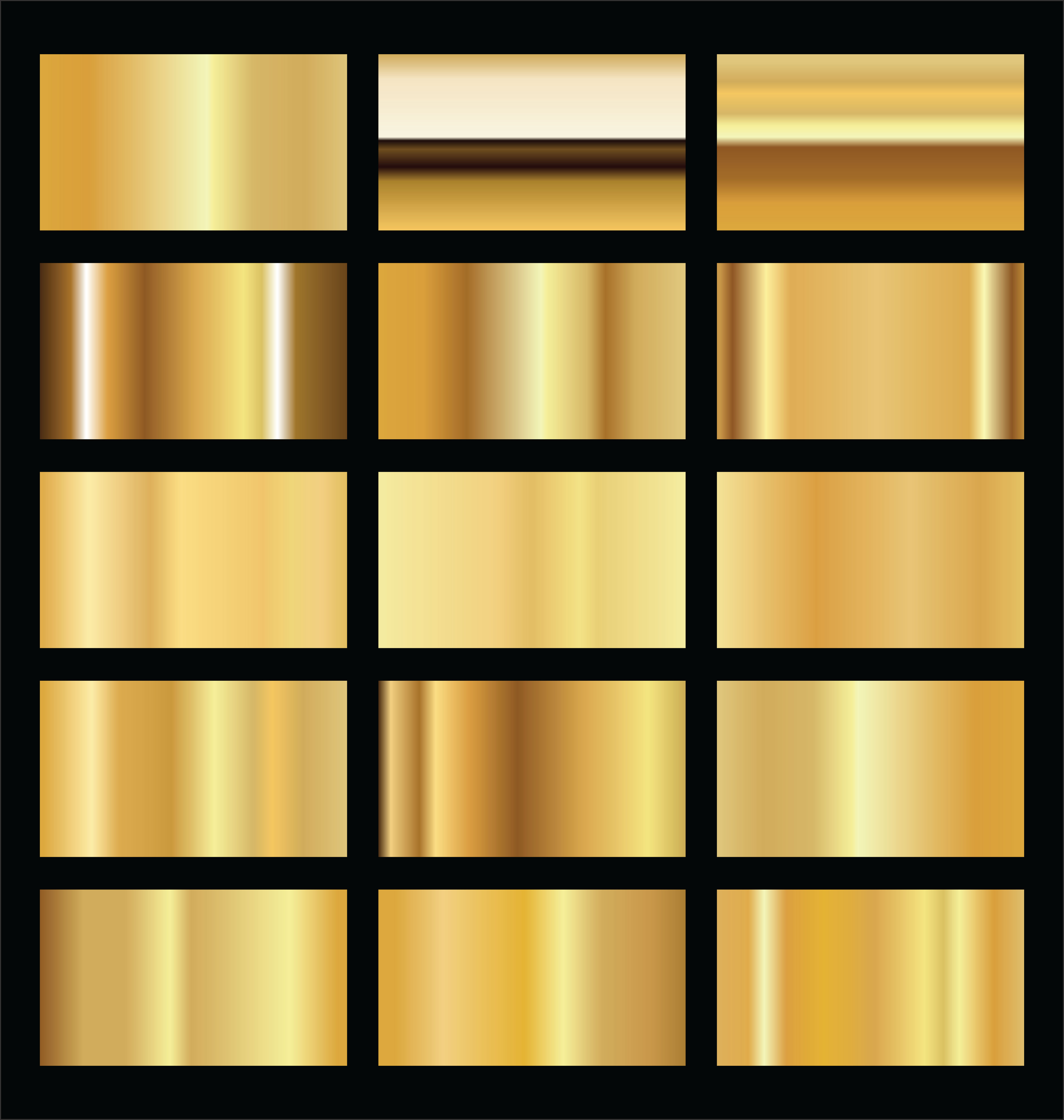
The only variation of the GANs with AAEs is that the latter controls the encoder output with the assistance of the prior distribution. The job of the autoencoders is to generate new random data with the help of given input data. propose another variation of the GANs called adversarial autoencoders (AAE) that converts an autoencoder into the generative model. Finally, we report an important observation, for the 32 × 32 × 32 grid presented here, that cache optimization is crucial for achieving parallel efficiency on the SGI Origin2000 machine. These subroutines contain data dependencies and will be addressed in a future publication.
#Gold vector code serial#
SPMD style OpenMP parallelization scales well for the 81 3 grid, but shows degradation due to the serial component in still unoptimized subroutines. Several arrays in csip5v.f are redefined for data locality, and computations are rearranged to optimize cache reuse.Īutomatic parallelization, PFA, scales comparably to SPMD style OpenMP parallelism, but performs poorly for larger scale sizes and when more than 8 processors are used. The FORTRAN subroutine is modified by changing the order of nested do loops so that the innermost index is the fastest changing index.
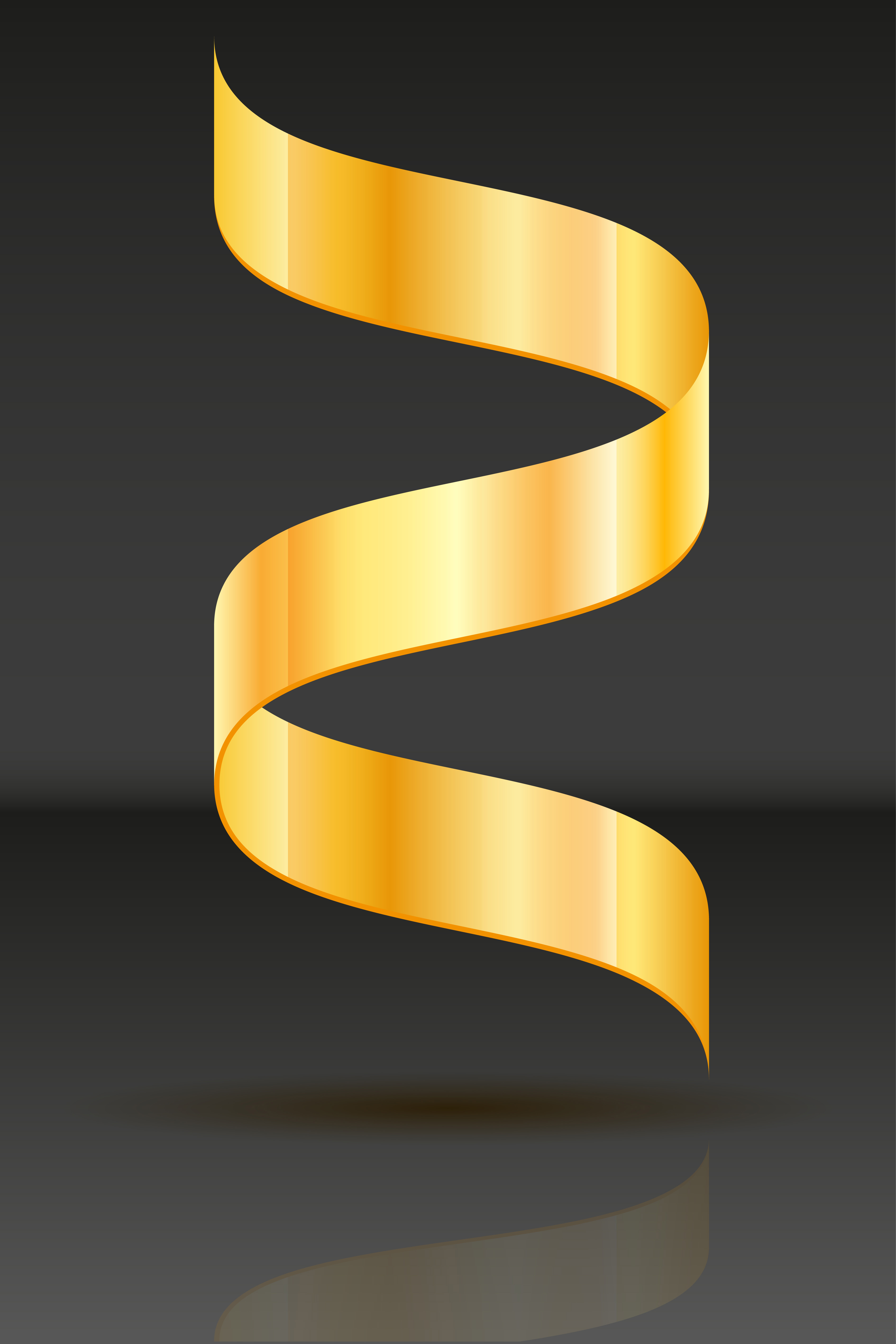
A significant portion of our time is spent in optimizing csip5v.f, an in-house LU decomposition solver, which happens to be the most expensive subroutine.
#Gold vector code code#
In summary, the CRAY C90/T90 vector code is optimized and parallelized for Ori-gin2000 performance. Richard Pletcher, in Parallel Computational Fluid Dynamics 1999, 2000 4 SUMMARY In many coding applications, such tradeoffs are often attractive. TSVQ is a popular example of a constrained quantizer that allows implementation speed to be traded for increased memory and a small loss in performance. For a larger (more realistic) codebook of size N = 256, the disparity is 8 versus 256, which is quite significant.
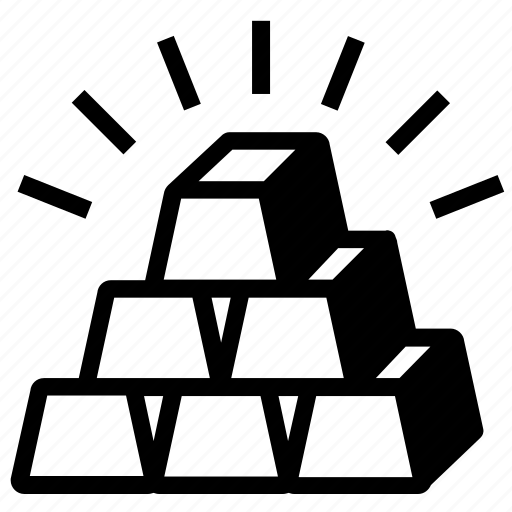
Implemented this way, only log 2 N distortion calculations are needed.įor the 8-vector TSVQ example above, this results in three instead of eight vector distortion calculations. The form of this calculation is the inner product between the hyperplane vector and input vector, where the sign of the output (+ or −) determines selection of either the right or left branch in the tree at that node. ), for each level, which results in only 2 log 2 N distortion calculations per input vector.Īlternatively, one can perform the decision calculation explicitly in terms of hyperplane partitioning between the intermediate code vectors.As presented, this implies the computation of two vector distortion calculations, d( For a binary tree, it is apparent that N = 2 v, which means that for a codebook of size N, only log 2 N decisions have to be made. After a decision is made at the first level, the same procedure is repeated for the next level until we have identified the codeword at the bottom of the tree. The first decision (at level v = 1) is to determine whether x is closer to vector y 0 or y 1 by performing a distortion calculation. During that process, we encounter v = 3 (or log 2 N) decision points (one at each level). To encode an input vector x, we start at the top and move to the bottom of the tree. In our particular example there are N = 8 codebook vectors and N = 8 paths in the tree, each leading to a different code vector. The search path to reach any node (i.e., to find a code vector) is shown explicitly in the tree. Are represented by the nodes at the bottom.
